Just Throwing It Out There is a 2x/month newsletter that provides deep thoughts on shallow things: fashion, luxury, eCommerce and the future of retail. If you enjoy this issue, subscribe below:
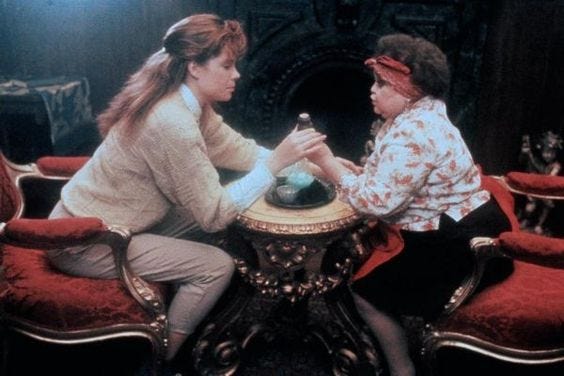
If you spend a lot of time on Reddit (and we all do, even if we won’t admit it), you’ve probably seen your fair share of time travel questions: “What would you do if you could time travel?”, “You wake up and find yourself in (some year in the past), what is the first thing you do?”
Inevitably, the top post is something about memorizing lottery numbers, buying Apple stock, or some other way to leverage current-day information to make money in the past. These responses expose the human fascination with predicting the future. It’s an obsession that is probably as old as time itself, and becomes stronger in times of uncertainty (like now!)
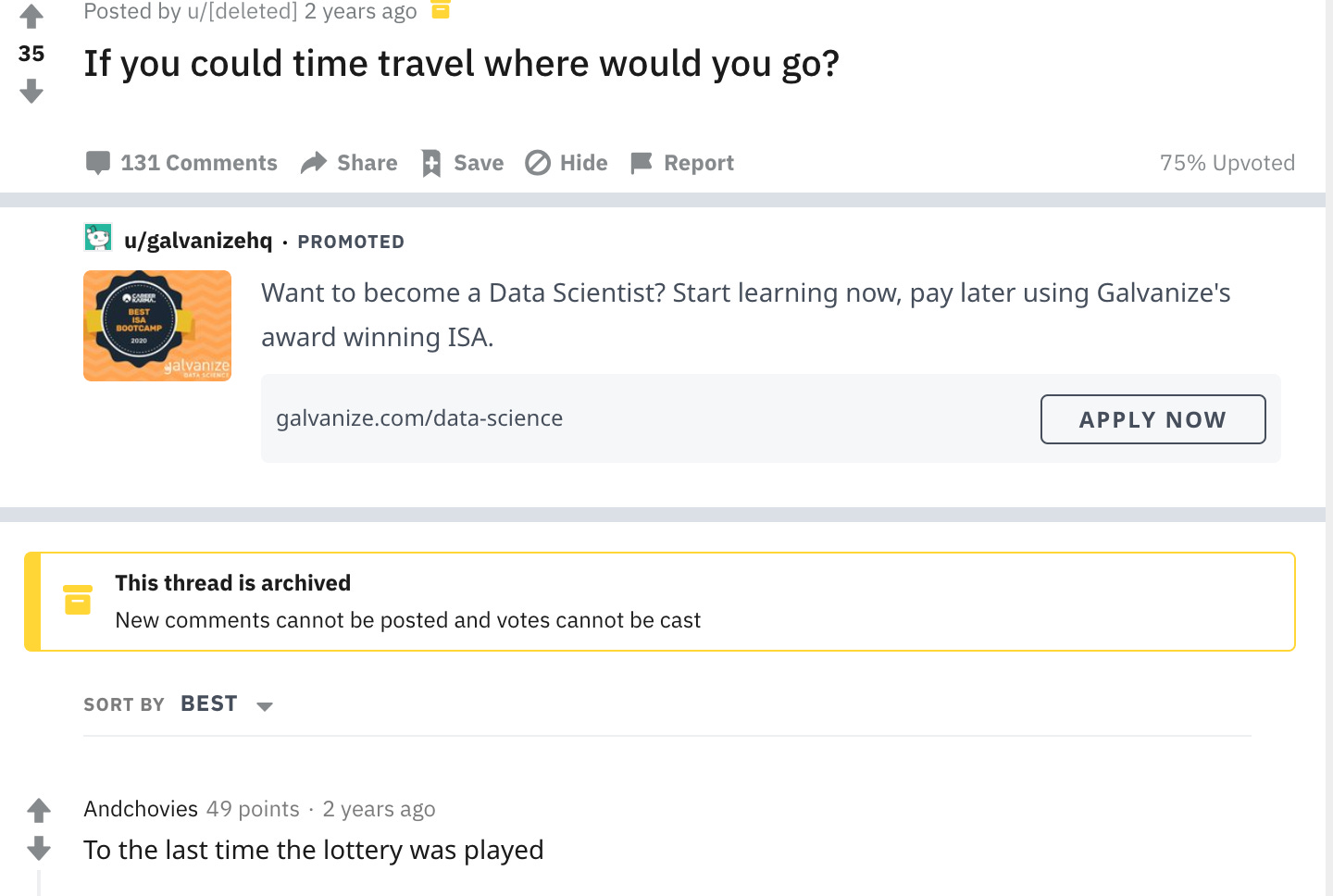
Whenever I observe something that a lot of people seem to want, or experience some sudden, intense desire for something, I try to stop and ask myself why I want this, or who is telling me I should want this, and why? So that’s what this newsletter is about: predicting the future.
That seems esoteric or fantastical. But stop for a minute and consider that you probably make your living from attempting to predict the future.
Outside of developing a rare set of useful skills, your salary or the value of your equity is tied to your ability to predict successfully. Small stakes predictions, small money. Big stakes predictions, big money, but also big potential downside.
Do I have your attention now?
The drive to predict the future is driven by fear or desire. We want to reduce uncertainty of bad outcomes, or we want to profit from knowing something that nobody else knows—two sides of the same nasty coin. But if everyone knew the future, that knowledge would no longer accomplish these goals. So what we’re really looking for is asymmetric information.
One of the best ways to understand the evolution of prediction is to study the practice of predicting the weather, and how it has changed over time. Predicting the weather isn’t stock picking—before the rise of global “industrial” agriculture, the weather was a matter of life and death. If weather patterns ruined the season’s harvest, many people would starve to death.
High level summary:
Ancient times: groups of people are geographically isolated and limited to observations that can be taken with the human body (five senses). We try to make sense of patterns between what we perceive and what happens later. Observations are subject to human error and difficult to store over time.
Ancient Greece: one smart guy thinks a lot, writes a book of observations about what causes the weather. A lot of it is wrong, but contains the “a ha” moment that weather is a product of the atmosphere, and not a whim of the gods.
Renaissance: people begin to think outside sensory data and invent instruments to more accurately measure things like air pressure. But the scope of what we decide to measure is still informed by what we’re able to observe.
Industrial Revolution: invention of the telegraph breaks down geographic barriers and allows groups of humans to collect and compile more data from more disparate places
Early 1900s: another “a ha” moment: we should try to develop mathematical models that describe atmospheric events that result in weather, which will make it possible to predict future weather
1940s onward: invent faster computers capable of storing and crunching more data to run and refine the models, and invent better tools for collecting more/better atmospheric observations
The outcome of this work is that a modern five-day weather forecast today is as accurate as a one-day forecast was in 1980. Our ability to predict the future means that famine is a thing of the past in most of the industrial world, major meteorological events kill fewer people, and I don’t ruin my blow-out as long as I remember to check my weather app in the morning.
The evolution of weather forecasting mirrors the general evolution of future-prediction in other sectors: figure out what is important to measure, invent instruments to measure it better, collect more observations until a rough equation emerges, invent tools to collect more/better observations and refine the model.
So why can’t we predict the future yet? Two answers to that question: (1) some things are more profitable to predict than others and (2) some things are harder to predict than others.
If you look at where the human race has applied the most energy to developing predictive infrastructure, financial markets come out on top. Financial markets present the challenge of working with a system impacted by human irrationality (“animal spirits”), but the market price of a financial instrument is also supposed to reflect everyone’s collected assumptions about its future value.
When you’re placing bets on the price of a financial instrument, predictive infrastructure is your only up-front cost. The only capital outlay is your position in the trade. You don’t have to build something to reap the reward, with all the associated uncertainty and risk.
Sectors that have seen a lot of investment in predictive infrastructure typically share these same characteristics. Another example: sports betting.
As the interest and the investment in predictive infrastructure within a sector increases, it becomes harder and harder for any given person to succeed; the “low hanging fruit” of asymmetric information gets competed away. You need to work to become the top 5-10% in the sector, and you need to specialize.
There are three ways to win:
(1) Invest in the development of more powerful predictive infrastructure. This could mean hiring the best talent to write your algorithms so they run better and faster. It could also mean investing in literal infrastructure—paying to lay your own cable between two geographic locations so that your trades reach the front of the queue faster.
(2) Look for new, more descriptive sources of information. This is what Google and Facebook are doing when they cultivate or purchase new audiences. They are not just looking for audience scale, but for new sources of data that may predict a person is ready to buy something. There is a reason these two companies are considered a duopoly in digital advertising—their ads work the best because they run off the best, most differentiated data on customer purchase intent. That differentiation enables them to invest heavily in #1 and #3 as well.
(3) sell the best picks and shovels
It’s interesting that the evolution of prediction roughly aligns with the evolution of cultural/artistic focus in the Western world.
Renaissance: deciding what to measure, inventing the first measuring tools, looking back to Ancient Greece for a jumping-off point.
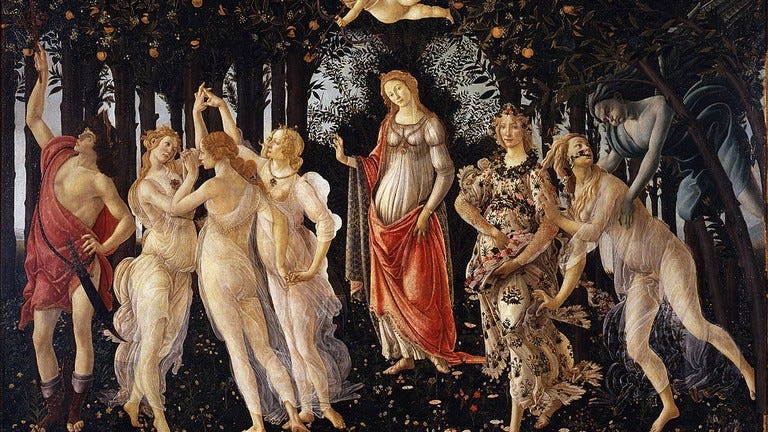
Industrial Revolution: inventing new/better measuring tools at scale, world getting smaller through travel and tech innovation (artists traveled for the first time, too).
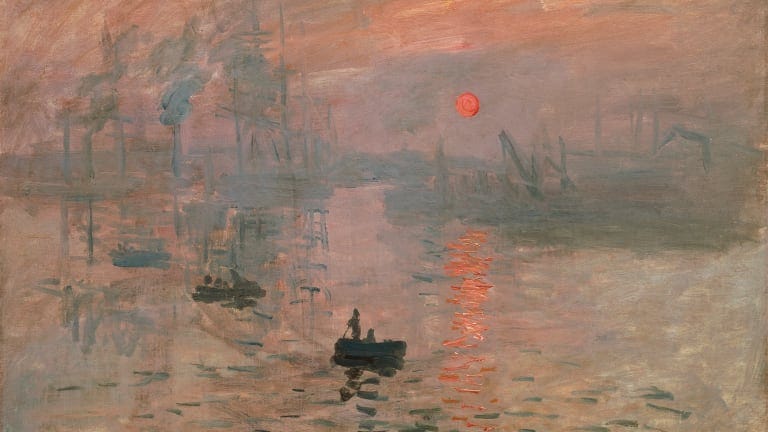
Early 1900’s onward (modernism): equations can predict the future, art moves away from narrative into abstraction.
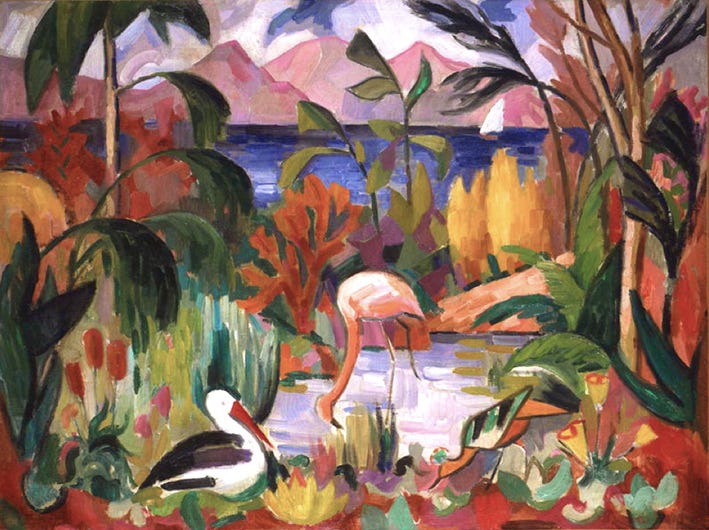
1950’s onward (post-modernism/contemporary art): working on behalf of computers, breaking down components and remixing, breaking down traditional definitions of what can and cannot be an input.
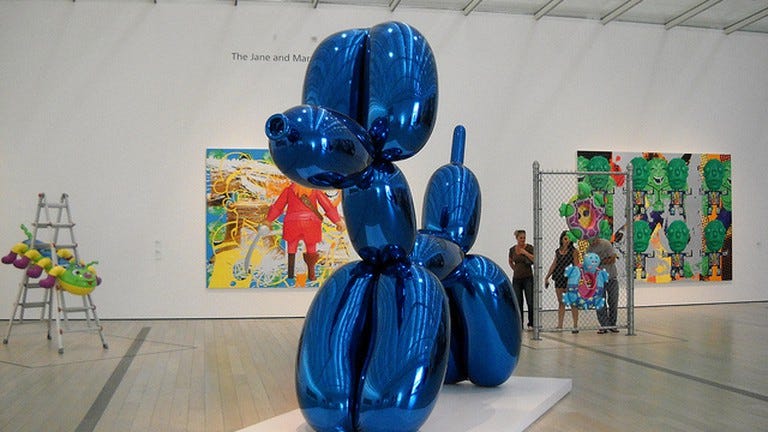
There is pretty solid proof that reality is shaped by the questions we choose to ask of it, and in the same way our own perspectives are shaped by the goals we choose to pursue.
What struck me about the “quest to predict the weather” is that it required international cooperation across generations to get us from rain dancing to weather satellites. There was an intergenerational agreement that predicting the weather was a worthwhile priority—not a formal agreement, but a shared understanding. Some people may have become wealthy or renowned as a byproduct of their work on the project, but it wasn’t the primary objective.
Looking at our current environment, our values, and where the most talented people are applying their time and energy—it’s not predicting the weather. Our current stage of prediction places value on breaking things into parts—how can we break the world down into causative factors, and then write equations that describe those factors? How can we overcome the “shortcomings” of human biology to make the computer work better?
It’s no surprise that as we shape our reality around these goals, it leads to a drought of imagination and a general feeling of being “stuck” or “alone”.
We have embarked on this project since roughly the post-WWII era, and our cultural focus has been mired in postmodernism for roughly the same amount of time. Postmodernism rejects certainty. This is a philosophy that aligns perfectly with the idea that the algorithm knows best, and our role in the whole thing is simply to engineer better inputs.
Technology has been accelerating so quickly that people are just beginning to wake up and question this. The individual decision between “going with the flow” of technological progress and mindfully turning away from it will define the next several decades of technology, government policy, consumer spending and culture.
In the interest of ~year end trendz~ pieces which are so popular on the internet, I will be sharing some specific predictions/implications for the future between now and the end of the year.
I’d love to make this more of a collaboration, so if you have hot takes on the future and want to talk about them (on the record or off), reply to this newsletter and let me know.
Additional Reading
Predicting The Weather: one, two, three
Gambling: one (paywalled), two
Picks & Shovels: one, two (paywalled)
Shaping Your Destiny: one, two, three
Header Image: Teen Witch